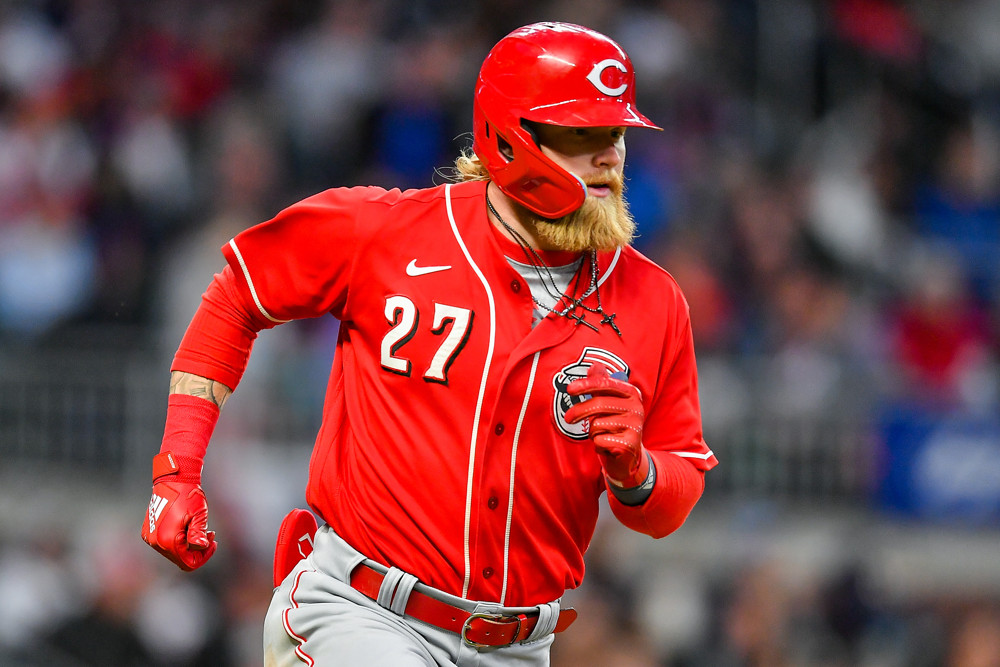
Thursday’s game marked the 12th game of the season for the Reds, and early next week, they’ll reach the 10% point for the season. The Reds’ offense currently checks in with a 90 wRC+, ranking 22nd in the Majors. Though sample sizes are still small and it’s important to remain cautious about drawing too many conclusions this early, Statcast metrics can provide some additional insight into hitters’ early success or struggles.
Here are the Statcast metrics for Reds hitters, prior to Thursday’s game.
For expected batting average (xBA), expected slugging percentage (xSLG), and expected weighted on base average (xwOBA), those highlighted in red indicate that the hitter’s expected metric is lower than the hitter’s actual production. For example, Jonathan India came into Thursday’s game hitting .310 and slugging .452, though based on his quality of contact, Statcast estimates that he should be hitting .259 with a .397 slugging percentage.
It’s a fairly even mix of players underperforming their Statcast metrics and players outperforming their Statcast metrics in the early going. A few in particular stand out, but perhaps none more so than TJ Friedl. Though he came into Thursday hitting .350 with a .600 slugging percentage, Statcast is not a big believer in his early success. A lot of this can likely be attributed to the fact that a few of those hits came in the form of bunts or otherwise weak contact in the infield. Still, Statcast doesn’t believe in the power he has shown either, with his .111 expected isolated power significantly trailing his .250 actual ISO.
Jason Vosler is another that jumps off the page. Though he’s cooled drastically from his hot start and his actual slash line reflects it, Statcast is even more pessimistic. Much like with Friedl, Statcast isn’t a big believer in his early power output.
On the other end of the spectrum, José Barrero stands out as someone that has underperformed the expected metrics thus far. For anyone that has been closely following the Reds’ games thus far, this likely isn’t a huge surprise to see. Barrero has had multiple cases of making hard contact where he was unable to reach base, and you can also see this in his abnormally low .222 batting average on balls in play (BABIP), often considered an indicator of “luck”. Barrero added a double and walk on Thursday to boost his slash line, and from what we have seen so far, there’s still some optimism that he may finally be starting to put it together.
Again, it must be cautioned that sample sizes are still small, and Statcast metrics don’t stabilize that quickly, so we must be careful with not drawing too many conclusions this early. A lot of analysis has been done into determining the stabilization rate for particular stats. Here are a few stabilization rates for hitters:
It’s important to note that no stat is ever actually fully stabilized. These are approximate points where a stat becomes stable enough where you can be confident in using it for analysis. As you can see, some stats will stabilize much quicker than others, and therefore provide more value in early season analysis. Others, like batting average and BABIP, don’t even reach their stabilization points in a single season, making it difficult for either of these to provide meaningful insights into a hitter.
Data-Driven Decision Making
A hot topic this week was David Bell’s decision to lift Jake Fraley for pinch hitter Kevin Newman in the eighth inning of a Tuesday loss to the Braves. The Braves had left handed reliever Dylan Lee on the mound, so Bell opted for the right handed Newman rather than the left handed Fraley, despite the fact that Fraley was 2 for 2 with a pair of walks in the game and is in the midst of a strong start to his season. The decision quickly backfired, with Newman striking out swinging.
Though the decision doesn’t look great in hindsight, and many are left saying “Fraley could have done that,” the saying goes that hindsight is always 20/20. While it is correct to say that Newman didn’t get the job done, and Fraley couldn’t have possibly done worse, that still doesn’t mean that Bell made the wrong decision. The truth is, we don’t know how Fraley would have done in that situation. He could have struck out himself, and then perhaps the conversation would have shifted to “why didn’t the Reds pinch hit for Fraley?”
Good decision making in this context relies on trusting the data. The data present in this particular situation was admittedly a bit muddy. It’s clear that Fraley has struggled in his career against LHP, slashing just .150/.259/.217 in 139 PA against LHP in the Majors. His .476 OPS in 31 PA against LHP last season didn’t show any meaningful improvement, and neither has his identical .476 OPS in 7 PA against LHP this season. One thing is clear, Fraley shouldn’t be facing LHP any more than he has to.
Where the data becomes more unclear is regarding Newman. He has a career 95 wRC+ against LHP, compared to a 69 wRC+ against RHP. He was even better against LHP last season, with a 140 wRC+ in 104 PA. In that regard, it seems like a no-brainer that you’d rather have Newman hitting against a LHP than Fraley. Digging deeper though, that may not be entirely the case. In 2021, Newman posted a 57 wRC+ against LHP in 177 PA. He was among the worst hitters in baseball in 2021 regardless whether there was a RHP or a LHP on the mound. Newman also has no future role with this team. He’s not a young, up-and-coming player that could be around for years to come. He’s an almost 30 year old backup infielder that’s more or less just a roster filler, and is just one year removed from being one of the worst players in the game.
The truth is, there are two entirely different questions in play here. The first is whether the Reds should trust the data and pinch hit for Fraley (and others) against LHP. The second is whether Newman is a good enough player to fill that role, and whether you’d rather give him plate appearances in any context rather than giving the chance to someone that may actually be a part of the Reds’ future plans.
The answer to the first question is overwhelmingly yes. Trusting the data goes a long way to having success in the game of baseball. There’s a reason that the Moneyball strategy was so widely praised. There’s a reason that teams are shifting more and more to trusting analytics and going strictly with the data. In order to keep up with the rest of the league, the Reds need to continue trusting the data. It may not always work out, and that’s perfectly fine. In the long run though, trusting the data is almost certain to work out more often than the other strategy, which in this context would be leaving Fraley in the game.
This whole situation looks a lot different if someone like Matt McLain was sent up there to fill this role, and it’s probably unlikely that there would be any sort of backlash. Hopefully we will reach the point this season where that is the case, and then David Bell can be left to truly trust the data without having to face backlash any time a decision doesn’t play in the Reds’ favor. In the meantime, Bell should continue to trust the data to give the Reds the best chance to win.
Even though there will undoubtedly be times where the decision does not pan out, the goal is just to end up with a net positive. As with all data, sample sizes matter. Looking at the success rate one decision, or even a handful of decisions, won’t provide meaningful insight into whether the decisions are working as intended. Rather, in order to analyze the net impacts of decision-making, a larger sample size over the course of the season is necessary. If data-driven decision making gets the Reds even five more runs throughout the course of any given season, it could be the difference between making the playoffs and missing out.
There’s a reason why teams like the Dodgers, Astros, and Rays have had so much success in recent years. While the Dodgers and Astros supplement the analytics focus with high payrolls, the same is not true of the Rays, who consistently have one of the lowest payrolls in the league. If the Reds want to compete without spending to the level of teams like the Dodgers, it becomes even more critical to squeeze as much value as possible out of the players on the roster. Making data-driven decisions is a key to that, as it allows the team to maximize production by putting players in the best position to succeed.
Featured Image: Twitter
Excellent article.
“Though the decision doesn’t look great in hindsight, and many are left saying “Fraley could have done that,” the saying goes that hindsight is always 20/20. While it is correct to say that Newman didn’t get the job done, and Fraley couldn’t have possibly done worse, that still doesn’t mean that Bell made the wrong decision. The truth is, we don’t know how Fraley would have done in that situation. He could have struck out himself, and then perhaps the conversation would have shifted to “why didn’t the Reds pinch hit for Fraley?”
Good decision making in this context relies on trusting the data. ”
“Resulting” and “association” rather than clear causation are classic mistakes. My only objection is that Hindsight is only sometimes 20/20. Often it’s, as I say, 20/40.
Problem with the average fan is that they take a results oriented approach when they judge Bell’s moves. Bell uses data to make his decision which he should be doing. Unfortunately, a pinch hitter only gets a hit approximately 33% of the time so most of the time the fans are going to grumble that Bell made the wrong move when he actually made the right call. Same with the bullpen. For some reason, fans expect the BP to have an ERA of zero so when a reliever inevitably gives up a run it’s because Bell picked the wrong guy to use.
100%